21st May 2019 –
Tests can be inconclusive.
And so all learning needs to go back into the testing mix and be used to come up with better and more refined ideas and hypotheses.
Which means, a whopping 78% of companies could optimize their optimization programs.
Once you start tapping into these sources, you should be able to generate a consistent stream of ideas.
And the companies running them look at the implementation of the experiments as the win. Their myopic view stops them from ever developing the infrastructure to support a consistent, quality testing program.
Because after you’re ready with all your good hypotheses, you need a way of scoring or prioritizing them. Doing so tells you which hypothesis to try first — or at all. HINT: “Let’s test a new website design!!! It’s going to shoot up our sales.” is usually a VERY BAD hypothesis.
What Kills Most Conversion Optimization Programs
By documenting its observations and learnings, LinkedIn was able to follow up on a failed experiment, which was actually a winner on the key feature being tested. Here’s the full scoop:
Focusing solely on execution and not spending enough time and effort on steps such as ideating, hypothesizing, and documenting/learning — that actually determine the quality of the experiments — usually results in only short-term success, if at all.
With a CRO tool like Compass (from our Convert Suite), you can effortlessly facilitate such data-backed, collaborative ideation. Compass lets you come up with data-backed testing ideas by bringing together your different data sources and also suggests ideas to test based on insights from Stuck Score™. Compass even lets you invite your team members and engage them with options for feedback and more.
In fact, only 22% of companies are happy with their CRO efforts.
For instance, take the famous 41 shades of blue experiment Google tried. Google’s experiment — data-informed as it was — was still criticized for taking an engineer-led approach. Here’s what Douglas Bowman, who worked as Google’s in-house designer, felt on how Google handled its experimentation: “Yes, it’s true that a team at Google couldn’t decide between two blues, so they’re testing 41 shades between each blue to see which one performs better. I had a recent debate over whether a border should be 3, 4 or 5 pixels wide, and was asked to prove my case. I can’t operate in an environment like that. I’ve grown tired of debating such minuscule design decisions.”
Typically, if you run 4 CRO tests each month (that’s a test/week), and if 10% of your tests win, you’re running a good optimization program. That’s a decent testing capacity and a nice win rate.
You might find it difficult to bring together all your data when ideating or suffer from data overwhelm when hypothesizing (and prioritization), or even struggle with documenting or using learnings for your follow up experiments, but these are the things that will help you increase testing velocity and set the groundwork for your long-term CRO success.
But most optimization programs don’t run so well.
How To Generate More Ideas to Test
Even better, if you manage a good uplift for your winning tests and your program’s performance keeps improving over time.
The team now decided to roll back to the original design by backtracking one change at a time, so that it could identify the one that didn’t go down well the users. During this time-consuming rollback, LinkedIn found that it wasn’t the unified search that people didn’t like, but it was a group of several small changes that had brought down the clicks and revenue. Once LinkedIn fixed these, unified search showed to have a positive user experience and was released for everyone.
You could also check out the PIE and ICE Score framework for prioritizing your hypotheses.
- Digging into the mountains of data your CRO tools generate. The best way of spotting testing ideas is to dig into your data. Your analytics solutions such as Google Analytics, Kissmetrics, Mixpanel, etc. are excellent sources for finding the pages where you lose most people or those that have low engagement rates. Tools like Hotjar, Clicktale, and Decibel show you what your users do on your website and can help identify your real conversion hotspots. Then there are solutions like UserTesting, UsabilityHub, and Usabilla among others that let you collect heaps of qualitative feedback that can translate to some crucial opportunities for testing. While it’s challenging to review so many data silos, these are the places where the real winning testing ideas come from.
- Running a manual CRO audit. Auditing your website for CRO uncovers some of the most valuable optimization gaps for testing. Running a CRO audit forces you to systematically look at each and every aspect of your website (and beyond) and see where you could be losing money.
- Using assessments like Stuck Score™ to spot the “conversion barriers” on your website. You can also use assessments like Stuck Score™ that uncover the conversion issues on your website and offer excellent ideas to test. These tools are intelligent and can precisely spot testing opportunities across your entire website.
An even smarter way of prioritizing your hypotheses is to use a CRO tool that can tell you how resource- and time-intensive an experiment can be. For example, Compass gives you good estimates for all your hypotheses.
To run (at least) 4 tests each month, you need a pipeline full of testing ideas. Without an “idea bank,” you can’t support a good and consistent testing velocity.
The problem with most optimization programs is that they aren’t designed for long-term success. Instead, they thrive on a test-by-test basis.
Of these, the ideas that can actually translate to strong hypotheses are your real testing opportunities.
By optimizing the ideating, hypothesizing, and learning parts of your CRO program, you can dramatically improve the quality of your experiments. And by collaborating and engaging with all your people over these, you can build and boost an all-inclusive culture of experimentation.
Forming Data-Backed Hypotheses and Laser-Focused Prioritization
Despite that, in most CRO programs, tests are planned when someone on the team has a CRO test epiphany of some sort.
For most conversion rate optimization programs, you get a low 20% of tests reaching statistical significance.
Compass helps you with data-backed ideation (by bringing together all the data from your different data silos and with inputs from Stuck Score™ suggesting ideas to try first), meaningful prioritization (by telling you how difficult, easy, or impactful an experiment could be), and the documentation of your learnings (by bringing together all your ideas, data research, observations, results, learnings and more in one place!).
Ideally, you should have a constant influx of quality testing ideas into your experimentation program. These testing ideas can come from:
But you need a LOT OF data to support each hypothesis you make. So for example, if you hypothesize that optimizing your mobile landing page experience will result in higher conversions, you’d need a bunch of data points to support it. In this case, here’s some of the data you could use:
- Low mobile conversions — data via your web analytics solution like Google Analytics.
- An unusually high dropoff for mobile traffic — again, data via your web analytics solution like Google Analytics.
- Poor feedback from customers — data via your user testing solution.
Here goes.
Some of your ideas would look promising and seem totally worthy of testing, but you’ll still need to look for “enough” data points to support them [more on this in a minute…].
And some ideas you’d simply have to discard because they’d be vague and you’d have no way to validate them. For example, if your CRO audit shows that you have a low NPS score, and you find it to be the reason for poor conversions, then you can’t possibly use a simple experiment to fix it.
So let’s see how you can increase testing velocity and run a good optimization program. If you’re already running one, you can use these tips to further improve your win rate and overall program performance.
While it’s true that good execution is a must for any experiment, but even a bad experiment can be executed really well.
But nobody wins when this happens.
Not just that, winning experiments can also be losers actually, when the challenger version wins but the revenue tanks.
Such programs are mostly only as effective (or not) as the last test they ran.
Learn From Your A/B Tests
Besides, if a hypothesis is a really strong and data-backed one, it’s common to create about 3-4 follow up experiments for it (even if the initial experiment won!).
But you’re not done yet.
Without sharing the ideas you’re considering and engaging your team, you can’t build an all-inclusive culture of experimentation that everyone wants to be part of.
Many factors go into deciding how practical testing a hypothesis is. Its implementation time and difficulty and the potential impact it can have on the conversions need to be considered here.
But how…
But most companies lack a prioritization model for this. This often results in the launching of an ambitious test like, say, a major design overhaul that uses up the entire month’s worth of CRO bandwidth. Which means you can’t plan or run anymore tests, at least for the month. The worst part is that even such ambitious tests don’t guarantee any significant results.
But the experiment failed and LinkedIn was surprised to see its key metrics tank.
Once you have your testing ideas, you’ll find that a few of them are just plain obvious. For example, if you get some user feedback that your content isn’t readable (and your target demographic are, say, people over forty years of age), then maybe you can implement the idea of increasing your font size or changing its color right away. After all, it’s a one-minute fix with a small CSS code change.
With a CRO tool like Convert Compass, you can build a knowledgebase of your ideas, observations, hypotheses, and learnings so your entire team can learn and grow together. Not just that, Compass can even use your learnings to suggest the hypothesis you could try next.
So whether it’s a simple A/B test or a complex multivariate one, any experiment you launch should be documented in detail. Its learnings need to be documented as well. By doing so, you can ensure that your future (or follow up) experiments are actually better than your earlier ones.
In 2013, LinkedIn Search kicked off a major experiment where it released its upgraded unified search functionality. Basically, LinkedIn Search got “smart enough” to figure out the query intent automatically without needed qualifiers like “People,” or “Jobs,” or “Companies.” The search landing page was totally revamped for this release — everything right from the navigation bar to the buttons and snippets were redone, so the users saw many, many changes.
Wrapping It Up…
But generating quality testing ideas is just one aspect of this issue. The other is the lack of communication and collaboration on the ideas under consideration. This might seem trivial (because, after all, you only need data, right?), but these issues deeply affect your people and can skew your culture of experimentation.
As you can see, the data to form this hypothesis is quite balanced as you’ve inputs from multiple data sources. Also, you’ve both quantitative and qualitative data. Ideally, you should find such balanced data to support all your “test-worthy” ideas.
If you’d rather use a CRO tool that does all this heavy-lifting for you, sign up below ⤵.
Which means, just interpreting and recording your experiment’s results isn’t enough. To plan meaningful iterative testing, you need to document your entire experimentation process each time you run one.
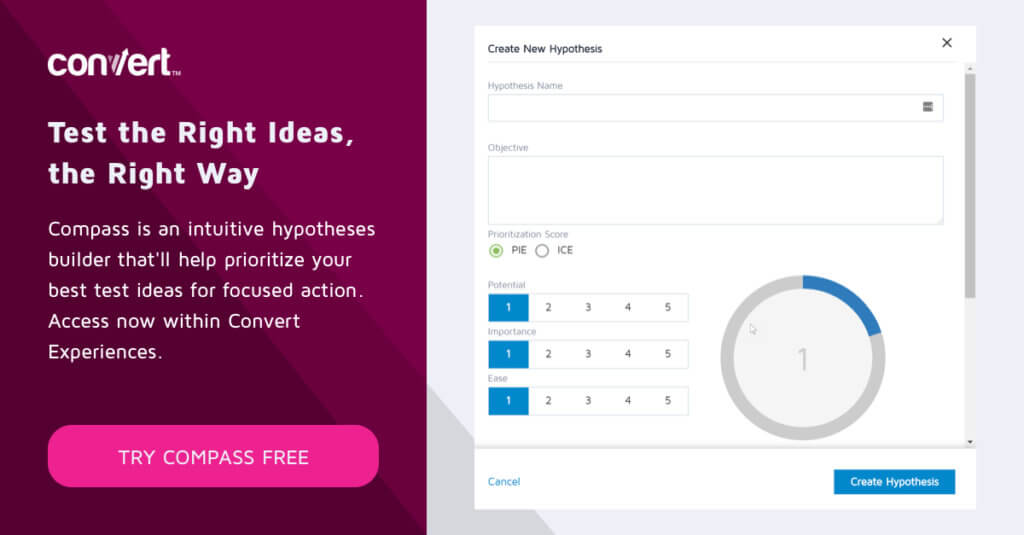
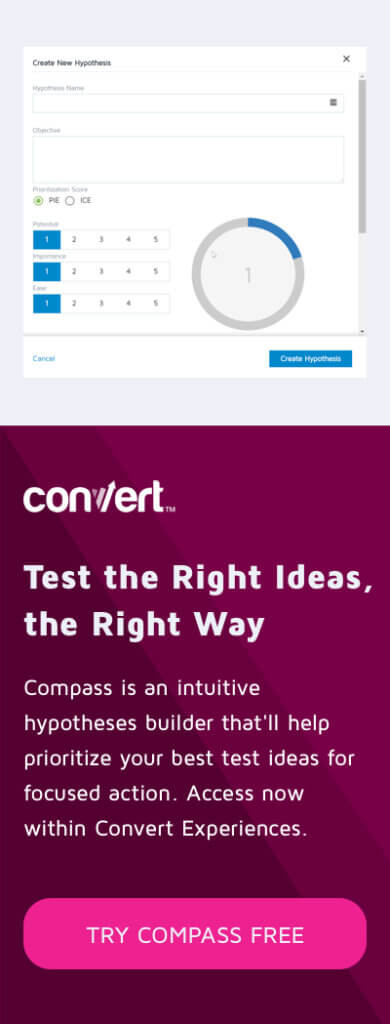